ML Parameters Optimization: GridSearch, Bayesian, Random
About this Course
Hello everyone and welcome to this new hands-on project on Machine Learning hyperparameters optimization. In this project, we will optimize machine learning regression models parameters using several techniques such as grid search, random search and Bayesian optimization. Hyperparameter optimization is a key step in developing machine learning models and it works by fine tuning ML models so they can optimally perform on a given dataset.Created by: Coursera Project Network
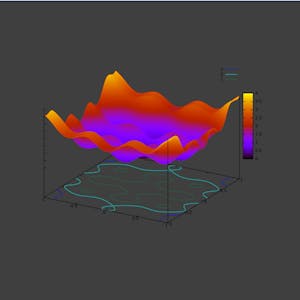
Related Online Courses
Gain the expertise needed to master Cisco\'s CCNP Security SNCF Exam (300-710) with this comprehensive course. You will develop advanced skills in deploying, managing, and troubleshooting Cisco... more
This course teaches you how to create stunning 3D game assets using Blender and Unreal Engine 5. Starting with the basics of Blender, you\'ll learn 3D modelling, texturing, and asset creation,... more
This course explores the topic of solid objects subjected to stress and strain. The methods taught in the course are used to predict the response of engineering structures to various types of... more
In Microsoft Azure, the Computer Vision cognitive service uses pre-trained models to analyze images, enabling software developers to easily build applications\"see\" the world and make sense of it.... more
This specialization will provide learners with the fundamentals and history of ESG investing, and a close examination of the set of investment approaches that are informed by environmental, social... more