ML Parameters Optimization: GridSearch, Bayesian, Random
About this Course
Hello everyone and welcome to this new hands-on project on Machine Learning hyperparameters optimization. In this project, we will optimize machine learning regression models parameters using several techniques such as grid search, random search and Bayesian optimization. Hyperparameter optimization is a key step in developing machine learning models and it works by fine tuning ML models so they can optimally perform on a given dataset.Created by: Coursera Project Network
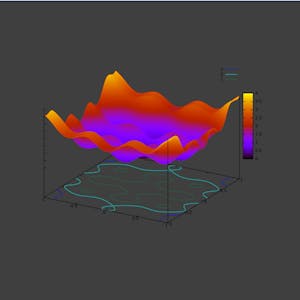
Related Online Courses
This course will cover various topics in Data Engineering in support of decision support systems, data analytics, data mining, machine learning, and artificial intelligence. You will study... more
This specialization is designed to teach learners beginning and intermediate concepts of statistical analysis using the Python programming language. Learners will learn where data come from, what... more
This comprehensive course equips you with skills to leverage Azure for building and deploying Large Language Model (LLM) applications. Learn to use Azure OpenAI Service for deploying LLMs,... more
In this course, we\'ll look at the JavaScript language, and how it supports the Object-Oriented pattern, with a focus on the unique aspect of how JavaScript approaches OO. We\'ll explore a brief... more
This course provides an in-depth understanding of how to leverage social listening to enhance customer engagement. It covers the foundational concepts and frameworks of social listening, its... more