Probabilistic Graphical Models 3: Learning
About this Course
Probabilistic graphical models (PGMs) are a rich framework for encoding probability distributions over complex domains: joint (multivariate) distributions over large numbers of random variables that interact with each other. These representations sit at the intersection of statistics and computer science, relying on concepts from probability theory, graph algorithms, machine learning, and more. They are the basis for the state-of-the-art methods in a wide variety of applications, such as medical diagnosis, image understanding, speech recognition, natural language processing, and many, many more. They are also a foundational tool in formulating many machine learning problems. This course is the third in a sequence of three. Following the first course, which focused on representation, and the second, which focused on inference, this course addresses the question of learning: how a PGM can be learned from a data set of examples. The course discusses the key problems of parameter estimation in both directed and undirected models, as well as the structure learning task for directed models. The (highly recommended) honors track contains two hands-on programming assignments, in which key routines of two commonly used learning algorithms are implemented and applied to a real-world problem.Created by: Stanford University
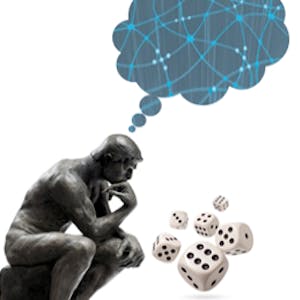
Related Online Courses
\"Generative AI for Business - A Leaders\' Handbook\" is a transformative course designed specifically for business leaders. This course demystifies the complex world of generative AI,... more
Data analytics is transforming the way businesses operate. But organizations often struggle with identifying opportunities for data-driven decision making and defining a clear analytics project... more
By its nature, the law touches on many aspects of entrepreneurship, making it an applicable and versatile topic of study. For aspiring entrepreneurs, it\'s important to understand how to form the... more
We have learned a lot recently about how the Universe evolved in 13.8 billion years since the Big Bang. More than 80% of matter in the Universe is mysterious Dark Matter, which made stars and... more
Learning Outcomes By the end of this course, learners will be able to: 1) Master the fundamentals and advanced features of ARM Cortex (STM32) microcontrollers. 2) Design and implement efficient... more