Decision Making and Reinforcement Learning
About this Course
This course is an introduction to sequential decision making and reinforcement learning. We start with a discussion of utility theory to learn how preferences can be represented and modeled for decision making. We first model simple decision problems as multi-armed bandit problems in and discuss several approaches to evaluate feedback. We will then model decision problems as finite Markov decision processes (MDPs), and discuss their solutions via dynamic programming algorithms. We touch on the notion of partial observability in real problems, modeled by POMDPs and then solved by online planning methods. Finally, we introduce the reinforcement learning problem and discuss two paradigms: Monte Carlo methods and temporal difference learning. We conclude the course by noting how the two paradigms lie on a spectrum of n-step temporal difference methods. An emphasis on algorithms and examples will be a key part of this course.Created by: Columbia University
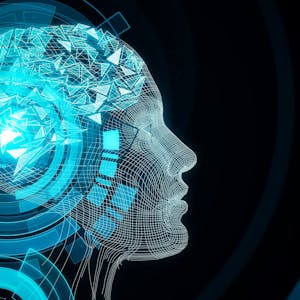
Related Online Courses
Recent events have once again put a spotlight on 400+ years of racism that has deeply entrenched our everyday structures, notably healthcare delivery. There is a significant need to provide a deep... more
This six course specialization is designed to prepare you to take the certification examination for IBM AI Enterprise Workflow V1 Data Science Specialist. IBM AI Enterprise Workflow is a... more
This course is designed for professionals interested in learning the principles of Lean Sigma, the DMAIC process and DFSS. This course is number 5 of 8 in this specialization dealing with topics in... more
Designed for learners of all experience levels, this program equips you with the essential skills needed to use the software tools utilized on a daily basis in office environments. Throughout the... more
In this course, you\'ll embark on a journey to master Apache Kafka, beginning with a solid introduction to its core concepts and architecture. You\'ll learn about essential topics such as... more