Explainable deep learning models for healthcare - CDSS 3
About this Course
This course will introduce the concepts of interpretability and explainability in machine learning applications. The learner will understand the difference between global, local, model-agnostic and model-specific explanations. State-of-the-art explainability methods such as Permutation Feature Importance (PFI), Local Interpretable Model-agnostic Explanations (LIME) and SHapley Additive exPlanation (SHAP) are explained and applied in time-series classification. Subsequently, model-specific explanations such as Class-Activation Mapping (CAM) and Gradient-Weighted CAM are explained and implemented. The learners will understand axiomatic attributions and why they are important. Finally, attention mechanisms are going to be incorporated after Recurrent Layers and the attention weights will be visualised to produce local explanations of the model.Created by: University of Glasgow
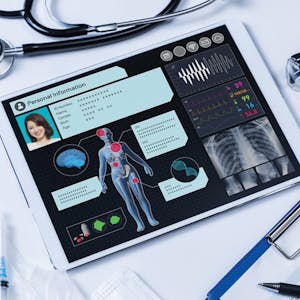
Related Online Courses
In this course you will be introduced to the basic ideas behind the qualitative research in social science. You will learn about data collection, description, analysis and interpretation in... more
H2O Wave Starter Course is meticulously designed for developers and AI enthusiasts eager to dive into H2O Wave, an open-source platform that simplifies the creation of browser-based AI applications... more
Welcome to Reigniting Employee Engagement with Professors J. Sanchez-Burks and Cheri Alexander. Engagement at work is tenuous at best. Stress and unhappiness remain elevated resulting in a myriad... more
Data structures play a central role in computer science and are the cornerstones of efficient algorithms. This specialization explores the principles and methods in the design and implementation of... more
This course, part 1 of a 2-course sequence, examines the history of rock, primarily as it unfolded in the United States, from the days before rock (pre-1955) to the end of the 1960s. This course... more