Decision Making and Reinforcement Learning
About this Course
This course is an introduction to sequential decision making and reinforcement learning. We start with a discussion of utility theory to learn how preferences can be represented and modeled for decision making. We first model simple decision problems as multi-armed bandit problems in and discuss several approaches to evaluate feedback. We will then model decision problems as finite Markov decision processes (MDPs), and discuss their solutions via dynamic programming algorithms. We touch on the notion of partial observability in real problems, modeled by POMDPs and then solved by online planning methods. Finally, we introduce the reinforcement learning problem and discuss two paradigms: Monte Carlo methods and temporal difference learning. We conclude the course by noting how the two paradigms lie on a spectrum of n-step temporal difference methods. An emphasis on algorithms and examples will be a key part of this course.Created by: Columbia University
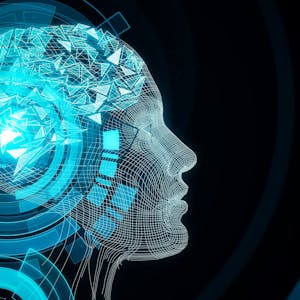
Related Online Courses
The purpose of this series of courses is to teach the basics of Computational Statistics for the purpose of performing inference to aspiring or new Data Scientists. This is not intended to be a... more
This specialization presents the first comprehensive treatment of the foundations of computer vision. It focuses on the mathematical and physical underpinnings of vision and has been designed for... more
Our course series on ICH Good Clinical Practice equips trial investigators and clinical researchers with the essential knowledge required to conduct reliable and ethical clinical trials. Whether... more
This 1.5 hours project-based course is the first part of \"Manage Your Versions with Git\". In this course, you will learn about the ubiquitous Git version management system. Git is an industry... more
This specialization is designed for post-graduate students aiming to advance their skills in AI, cybersecurity, & industrial control systems (ICS) security. Through three in-depth courses, you will... more